Azure Kubernetes Service provides powerful automation and scaling features; however, inefficient resource allocation, over-provisioning, and unused capacity can lead to unnecessary spending.
In this article, you’ll learn about AKS, understand its pricing and cost components, and best Practices for AKS Cost Optimization.
Let’s dive in!
What is Azure Kubernetes Service (AKS)?
Azure Kubernetes Service (AKS) is a managed Kubernetes service from Microsoft Azure that simplifies deploying, managing, and scaling containerized applications. It provides a free control plane, automated updates, and built-in security while handling complex Kubernetes operations for users.
Understanding AKS Pricing & Cost Components

Let’s understand the AKS pricing components and how billing works:
AKS provides a free control plane, meaning you only pay for the worker nodes running your workloads. But the costs come from various factors like compute costs, ctorage costs and networking costs. The different VM sizes, node scaling, storage and network usage directly impact your costs.
In AKS, the billing depends on various SKU (Stock Keeping Unit) types and pricing factors:
- Compute SKUs: It is based on VM type and pricing model.
- Storage SKUs: It depends on the type of Azure disks used.
- Networking SKUs: It covers the load balancers and data transfer costs.
Azure Kubernetes Service (AKS) provides different SKU (Stock Keeping Unit) types for its compute, storage, and networking components. These SKUs define the pricing model and capabilities available to users.
Let’s understand these SKUs:
1. Control Plane Costs (Free vs. Paid)
Free Control Plane: AKS provides a managed control plane at no cost, meaning you don’t pay for Kubernetes API servers or master nodes.
Paid Control Plane: Azure provides a Uptime SLA (Service Level Agreement) for production-critical workloads, where you pay for a guaranteed uptime SLA for the control plane.
2. Node Costs (Compute SKUs)
Compute resources in AKS are the biggest cost factor. The main SKU types for AKS compute instances include:
On-Demand VMs: It provides standard pricing with full flexibility but at a higher cost.
Spot VMs: It is of lower-cost, provide interruptible instances, that is ideal for fault-tolerant workloads.
Reserved Instances (RI): It provides discounted pricing for long-term commitments (1 or 3 years).
Savings Plan for Compute: It is a flexible commitment-based pricing model that applies discounts across multiple instance types.
3. Storage SKUs
Storage costs depend on the type of persistent volumes and disks used:
Premium SSDs: provide high-performance, low-latency storage and are best for critical applications.
Standard SSDs: provide a balance between performance and cost for general workloads.
Standard HDDs: is the cheapest option for infrequent access workloads.
Azure Blob Storage: cost-effective object storage for backups and logs.
4. Networking Costs (Load Balancers & Data Transfer)
Network costs come from:
Load Balancers: AKS provides Standard Load Balancers (paid, better security) and Basic Load Balancers (free but with limited features).
Data Egress: When traffic leaves Azure (cross-region or internet-bound), it incurs costs.
Best Practices for AKS Cost Optimization
Here are some of the best practices that you can follow:
1. Compute Optimization
2. Scaling & Workload Optimization
3. Networking Cost Optimization
4. Storage & Persistent Volume Optimization in AKS
Now, let’s discuss these best practices one by one in detail:
1. Compute Optimization
a. Choosing the Right Node Size
You should select the right node size, which affects both performance and cost efficiency. The key factors to consider are small vs. large nodes and CPU vs. memory-bound workloads. Let’s discuss:
Small vs. Large Nodes: One of the biggest decisions in AKS optimization is choosing between many small nodes or fewer large nodes. Each approach has its own advantages and disadvantages:
Small Nodes: Small Nodes (e.g., D2s_v4, B-series) work well for dynamic applications that require frequent scaling, such as web applications and microservices. They allow for more precise autoscaling, ensuring that resources are allocated efficiently and minimizing waste. But having many small nodes can introduce network overhead due to increased inter-node communication.
Large Nodes: Large Nodes (e.g., D16s_v4, F-series, E-series) are better suited for high-performance workloads such as databases, AI model training, or large-scale batch processing. With fewer nodes, the operational overhead per node decreases, reducing the burden on kube-proxy, monitoring agents, and other background processes. Large nodes also improve network efficiency by minimizing inter-node communication. But they can lead to resource fragmentation, making it difficult to schedule small workloads effectively, as leftover resources may not always align with new workload demands.
The best approach is to use a combination of both small and large nodes based on workload characteristics. Large nodes should be reserved for resource-intensive applications that require high performance and stability, while small nodes can handle workloads that need rapid scaling resulting in cost efficiency and better resource management.
b. CPU vs. Memory-Bound Workloads
You should select the right VM types based on your workload if it’s CPU-intensive and memory-intensive.
CPU-intensive Workloads: CPU-intensive workloads like AI processing, game servers, and video encoding, require high clock speeds and multiple cores to process tasks efficiently. These workloads perform best on compute-optimized nodes like the F-series in Azure. Since CPU-heavy workloads can tolerate interruptions, they can be deployed on Spot VMs to take advantage of lower pricing while maintaining cost efficiency.
Memory-Intensive Workloads: Memory-intensive workloads, such as databases, caching systems, and data analytics applications, require higher RAM per vCPU to manage large datasets. These workloads are running well if we choose memory-optimized nodes, such as the E-series in Azure, which provide ample memory for handling data-intensive operations.
If you want to maximize cost efficiency, it’s important to match each workload’s compute requirements with the appropriate node type. By carefully selecting node types based on workload demands, organizations can minimize waste and optimize their AKS infrastructure.
c. Using Spot VMs & Reserved Instances for Savings
Azure Spot VMs let you tap into unused Azure capacity at a steep discount sometimes up to 90% off regular prices. They work best for flexible, interruption-tolerant workloads such as batch processing, CI/CD pipelines, AI model training, and even web applications. Because these VMs can be reclaimed at any moment by Azure, it's important to have fallback plans in place. This could mean using Pod Disruption Budgets or backup nodes to keep your applications running smoothly even if a Spot VM goes offline.
For workloads that need to run without interruption, Reserved Instances are the go-to choice. They provide savings of up to 72% compared to on-demand pricing and are perfect for databases, APIs, persistent services, or any application with steady demand.
By combining both options, use Spot VMs for tasks that can handle interruptions and Reserved Instances for critical applications, you can create a balanced, cost-effective strategy that maximizes savings.
>> Take a look at Amazon EKS Cost Optimization Best Practices
d. Node Pool Optimization
You should segment your AKS cluster into multiple node pools, which helps enhance cost efficiency and workload management. By creating dedicated node pools for specific types of tasks, you ensure that each workload runs on hardware tailored to its needs. For example, a general-purpose node pool works well for web applications and APIs, while memory-optimized node pools are ideal for databases and in-memory caching tasks. Compute-optimized node pools are perfect for high-demand processes like AI/ML workloads and batch jobs. Also, having a dedicated spot node pool for non-critical and flexible workloads allows you to take advantage of cost savings without compromising on performance where it's most needed.
You can also use AKS Node Auto-Provisioning to further refine this strategy by automatically creating node pools as demand increases. This means your cluster can dynamically adjust to changing workload patterns without manual intervention, making sure that you always have the right type of nodes available while avoiding over-provisioning.
2. Scaling & Workload Optimization
a. Cluster Autoscaler, HPA, KEDA & Karpenter
The default Cluster Autoscaler adds and removes nodes based on resource needs, but it only retires nodes that are completely empty. This limitation means that nodes with some workload, even if underutilized, continue to run and contribute to costs. Karpenter offers a better approach by actively assessing incoming pod demands and provisioning nodes that exactly match those requirements. It also has the ability to quickly remove nodes that aren’t fully used and helps in reducing expenses. If you have environments with fluctuating workloads, switching to Karpenter can provide faster, more cost-efficient scaling. On AKS managed Karpenter service is available in preview as NAP (Node Auto Provisioning) or can be self-installed from https://github.com/Azure/karpenter-provider-azure
The Horizontal Pod Autoscaler (HPA) scales the number of running pod replicas based on CPU or memory usage. It ensures that applications get the right amount of resources without overloading nodes. HPA is best suited for steady, predictable workloads where traffic increases gradually.
The workloads that are driven by unpredictable events, such as sudden spikes in API requests or queue-based processing, KEDA (Kubernetes Event-Driven Autoscaler) provides better efficiency in this case. Unlike HPA, which relies only on CPU and memory metrics, KEDA scales workloads based on external events such as messages in a Kafka queue, database load, or cloud-based events like Azure Functions. By scaling workloads only when needed, KEDA reduces idle resource costs.
b. Using Vertical Pod Autoscaler (VPA) to Reduce Overprovisioning
Vertical Pod Autoscaler (VPA) helps to avoid overprovisioning resources by automatically adjusting the CPU and memory requests of running pods based on actual usage patterns.
Instead of manually setting resource requests and limits, VPA analyzes historical usage and dynamically updates the values to match real demand. This prevents pods from consuming unnecessary resources while ensuring they get enough capacity during peak loads. For cost-sensitive applications, you should enable VPA in recommendation mode, which allows teams to monitor optimizations before applying them in production.
>> Learn more about the Kubernetes Vertical Pod Autoscaler
c. Right-Sizing Requests & Limits
You should set the right CPU and memory requests, which is important for efficient workload management. If requests are too high, nodes remain underutilized, leading to increased cloud costs. If requests are too low, applications may suffer from performance issues due to throttling or out-of-memory errors. The best practice is to analyze actual resource consumption and fine-tune requests and limits accordingly.
You can use tools like kubectl top, Metrics Server, and Prometheus to help monitor resource usage, while Kubernetes-native features like VPA assist in auto-tuning requests over time.
And if you are using the PerfectScale platform for your cluster visibility, you can just go to the alerts tab and quickly identify the errors resulting from your Kubernetes resource misconfigurations and resolve them quickly.

3. Networking Cost Optimization
a. Load Balancer & Ingress Controller
The right load balancer and ingress controller totally impacts your performance and costs.
Standard vs. Basic Load Balancer: Azure provides two tiers of load balancers, i.e., Standard and Basic. The Standard Load Balancer provides features like higher availability, more backend pool capacity, and support for availability zones. While it incurs higher costs compared to the Basic tier, its advanced capabilities can justify the investment for production environments requiring robust performance and reliability.
NGINX vs. Azure Application Gateway: When you want to choose an ingress controller, NGINX and Azure Application Gateway are popular options. NGINX is a versatile, open-source solution that offers flexibility and extensive customization. On the other hand, Azure Application Gateway is a managed service that integrates with other Azure offerings, providing features like built-in WAF (Web Application Firewall) and SSL termination. While Azure Application Gateway may have higher associated costs, its managed nature can reduce operational overhead.
b. Reducing Data Egress Costs
Private Endpoints & Peering: You should implement private endpoints that allow resources within your virtual network to communicate securely and privately, which eliminates the need for public IP addresses. This setup not only increases security but also reduces data transfer costs associated with public endpoints. Similarly, virtual network peering enables direct, low-latency connectivity between virtual networks, providing efficient data flow without incurring additional egress charges.
Minimizing Cross-Region Traffic: The data transfers between Azure regions can lead to costs. To minimize these expenses, deploy resources within the same region whenever possible. You should align your AKS clusters, storage accounts, and other services geographically to ensure that data remains within a single region, thereby reducing cross-region data transfer fees.
4. Storage & Persistent Volume Optimization in AKS
a. Choosing the Right Azure Disks & Storage Classes
Azure provides multiple disk and storage options, and selecting the right one for your workload is key to cost savings:
Managed Disks: Azure offers Premium SSDs, Standard SSDs, and Standard HDDs. Each has different performance and cost trade-offs.
Storage Classes in AKS: The default storage class in AKS provisions Premium SSDs, which might not always be necessary. You should choose standard SSDs or HDDs for non-critical workloads, which can cut costs.
Ephemeral Disks: If your application doesn’t require persistent storage, using ephemeral OS disks instead of managed disks can reduce costs and improve performance.
b. Premium SSDs vs. Standard HDDs
Azure Premium SSDs deliver low latency and high IOPS; they're good for performance-sensitive workloads. But they are expensive. If your applications don’t require high throughput, you should use standard SSDs or HDDs, which can save costs without noticeable performance degradation.
When to choose each option:
Premium SSDs: You should use these for critical applications, databases, or workloads requiring high IOPS and low latency.
Standard SSDs: It’s suitable for general-purpose workloads where moderate disk performance is acceptable.
Standard HDDs: It's best for archival storage, backup, and applications with low read/write requirements.
c. Using Azure Blob Storage
For unstructured data, Azure Blob Storage is a cheaper alternative to managed disks. Instead of attaching persistent volumes for logs, backups, or static assets, you should store them in Azure Blob Storage, which can help in cutting down storage expenses.
Hot vs. Cool vs. Archive Tiers: Blob Storage has different access tiers:
- Hot Tier: It is best for frequently accessed data.
- Cool Tier: It is more cost-effective for infrequently accessed data.
- Archive Tier: It is the lowest-cost option for long-term storage of rarely accessed data.
Using Azure Files: If you need shared storage across multiple pods, Azure Files (especially Standard tier) can be a cost-effective alternative to persistent disks.
d. Backup & Disaster Recovery Cost Considerations
Backup and disaster recovery are important but can be optimized for cost:
Azure Backup vs. Custom Solutions: While Azure Backup is easy to use, it can be costly. The custom solutions like Velero or Restic allow more control over retention policies and cost savings.
Snapshot Optimization: Instead of keeping multiple full backups, incremental snapshots can reduce storage costs while providing data safety.
Cross-region replication costs: If you replicate data across regions, be mindful of data transfer costs. You should keep backup copies within the same region to reduce expenses.
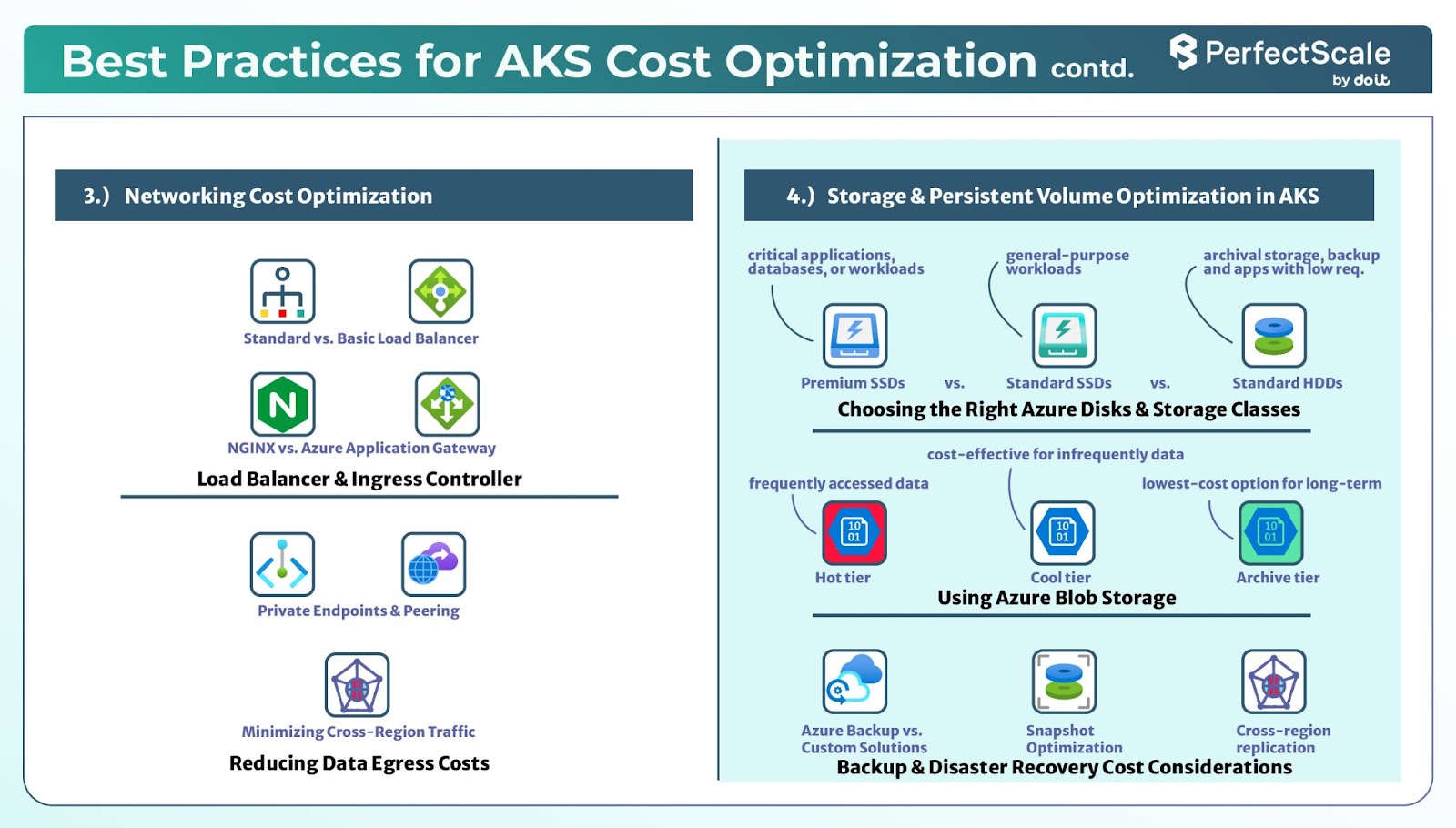
How PerfectScale Can Elevate Your AKS Cost Management
Integrating PerfectScale into your AKS strategy ensures that your Kubernetes environment remains both cost-efficient and high-performing. PerfectScale continuously monitors your workloads, detecting underutilized resources and potential inefficiencies, then applies automated right-sizing to align resource allocation with actual demand. This proactive optimization eliminates waste and ensures that your AKS clusters are always running at peak efficiency. Additionally, PerfectScale provides granular insights into node utilization, helping you make informed decisions about resource scaling and instance selection. By leveraging PerfectScale, you gain a streamlined, intelligent approach to AKS cost management reducing expenses while maintaining reliability and performance. To experience the benefits of PerfectScale firsthand, consider Booking a Demo today and Start a Free Trial today!
